AdaBoost决策树回归¶
使用AdaBoost提升决策树。在一维正弦数据集上的R2[1]算法,具有少量高斯噪声。299个增强(300个决策树)与一个决策树回归器进行比较。随着提升次数的增加,回归器可以适应更多的细节。
1Drucker, “Improving Regressors using Boosting Techniques”, 1997.
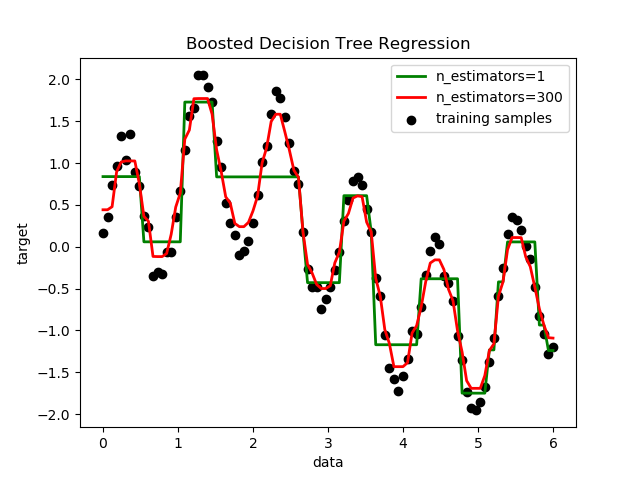
print(__doc__)
# Author: Noel Dawe <noel.dawe@gmail.com>
#
# License: BSD 3 clause
# importing necessary libraries
import numpy as np
import matplotlib.pyplot as plt
from sklearn.tree import DecisionTreeRegressor
from sklearn.ensemble import AdaBoostRegressor
# Create the dataset
rng = np.random.RandomState(1)
X = np.linspace(0, 6, 100)[:, np.newaxis]
y = np.sin(X).ravel() + np.sin(6 * X).ravel() + rng.normal(0, 0.1, X.shape[0])
# Fit regression model
regr_1 = DecisionTreeRegressor(max_depth=4)
regr_2 = AdaBoostRegressor(DecisionTreeRegressor(max_depth=4),
n_estimators=300, random_state=rng)
regr_1.fit(X, y)
regr_2.fit(X, y)
# Predict
y_1 = regr_1.predict(X)
y_2 = regr_2.predict(X)
# Plot the results
plt.figure()
plt.scatter(X, y, c="k", label="training samples")
plt.plot(X, y_1, c="g", label="n_estimators=1", linewidth=2)
plt.plot(X, y_2, c="r", label="n_estimators=300", linewidth=2)
plt.xlabel("data")
plt.ylabel("target")
plt.title("Boosted Decision Tree Regression")
plt.legend()
plt.show()
脚本的总运行时间:(0分0.477秒)